Summary
Dr. Felipe A. Medeiros discusses AI's use in glaucoma clinical settings
~Doctor, I Have a Question. How prevalent is the use of AI in glaucoma clinical practices today? ~
Question answered by:
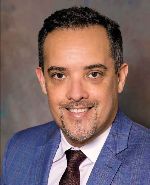
Professor of Ophthalmology
Vice Chair of Research
Bascom Palmer Eye Institute, FL
Although there have been a lot of developments in AI, bringing them to real-world glaucoma clinical settings has actually been quite slow.
One reason for the complexity in implementing AI in medical practice is the extensive regulatory framework. For instance, considerable research has focused on enhancing optical coherence tomography (OCT) imaging for glaucoma diagnosis using AI, including work conducted in my lab and others. However, beyond developing these AI models, there are additional steps required for clinical integration. To deploy these models on diagnostic devices, companies must conduct comprehensive clinical trials to meet FDA requirements, ensuring the algorithms are both safe and effective.
That’s understandable, as regulatory bodies are fulfilling their oversight responsibilities. However, to date, only a few ophthalmologic AI applications have received approval, and notably, none of these pertain directly to glaucoma.
As we continue to rely on traditional methods such as electronic medical records, standard OCT imaging analyses, and long-established visual field testing techniques, the transformative potential of AI in clinical practice remains largely untapped. Despite significant advancements and the promising capabilities of AI, the integration into daily medical practice is progressing slowly due to various constraints.
One area where AI is being adopted more rapidly is in quality assessment and improvement. Much like AI enhances photo quality on smartphones, it can also enhance the quality of images and tests in devices used for glaucoma assessment. The regulatory requirements for such supportive applications tend to be less stringent than those for direct diagnostic tools, facilitating faster integration into clinical practice.
For example, when you acquire an image of your retina with OCT for the purpose of evaluation for glaucoma, you are interested in looking at specific features, like measuring the thickness of the nerve fiber layer. The OCT software must then segment the different layers of the retina to obtain such thickness measurements. This can be done much better with AI compared to the older algorithms.
There is also a lot of work being done in the area of screening, trying to come up with AI that could be made widely available – for example based on simple photographs of the back of the eye to identify glaucoma. While there are commercially available machines approved by the FDA that can be used for screening of diabetic retinopathy, this has proven harder for glaucoma.
One of AI’s strengths lies in its ability to identify patterns, particularly useful in interpreting images and visual field tests for glaucoma management. Typically, a clinician assesses a patient by measuring intraocular pressure, examining the optic nerve, and reviewing OCT and visual field test results. Integrating all this data to diagnose glaucoma and determine disease progression can be complex. AI can streamline this process by analyzing the data more objectively, enhancing the precision and efficiency of glaucoma diagnosis and monitoring.
While there is ongoing progress in developing these decision-support systems, and their promise is undeniable, several challenges still need to be overcome before AI can be fully integrated and adopted into clinical practice. The process is underway, but it will take time.